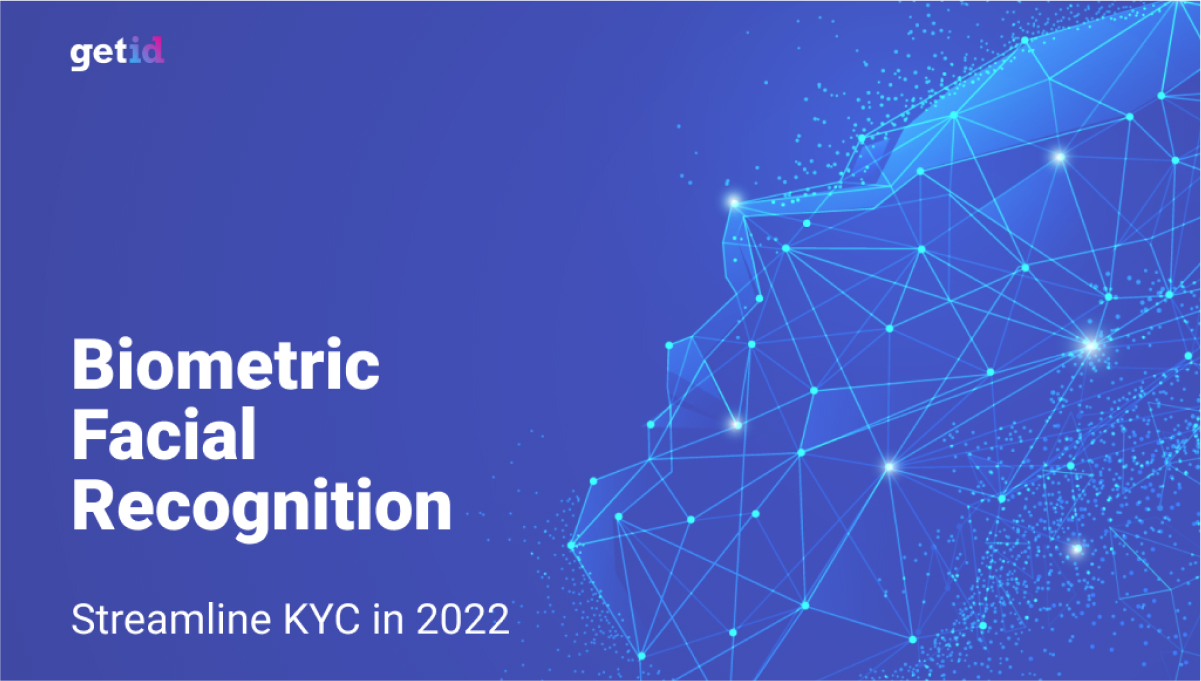
How to use Biometric Facial Recognition to streamline KYC in 2023
11 Jan 2022
Introduction
In a world where online fraud and digital hacks are on the rise, it’s never been more important to verify that your customers are who they say they are.
Luckily, advancements in facial recognition software now enable streamlined biometric identification and authentication. For you, this means KYC and AML onboarding processes.
Harnessing the power of GetID’s online identity verification platform offers your company the chance to deploy biometric facial recognition and strengthen your identity verification processes.
Like all next-generation technologies, biometric facial recognition is a double-edged sword.
Advanced applications of facial recognition, like GetID, can help to identify and authenticate users faster and is more cost-effective. However, picking the wrong facial recognition service can leave your company facing unexpected challenges. This is why questions concerning data security, privacy, and misidentification have come to the forefront of facial recognition discussions.
Fortunately, GetID stays ahead of the curve when it comes to keeping users secure and compliant. Not only does our deployment of biometric facial recognition technology eradicate friction in your customer onboarding process, but its robust architecture and automated workflow also keeps your company compliant with all AML and data security regulations.
But let’s start at the beginning…
What is Biometric Facial Recognition?
Biometric identification technology encompasses software that identifies persons by their biological characteristics. For example, criminal investigations may use biometric fingerprint or DNA scanners, while high-tech security systems may involve iris scanning technology. Biometric facial recognition software identifies people from their facial features.
Most often, biometric facial recognition is used to automatically recognize a person from their face, a video, or an image. This is usually for biometric authentication, identification, or categorization.
As facial recognition technology scans the patterns in images or faces to find a match, it could be classed as a visual pattern recognition problem. The technology reconstructs a three-dimensional image from the information on a two-dimensional image to match that image to a person.
Using lighting, expressions, and poses, biometric facial recognition can match the visual patterns between the two-dimensional image and the three-dimensional person. Biometric facial recognition technologies tend to deploy five modules to complete this task: detection, normalization, processing, feature extraction, and matching.
The reason that biometric facial recognition has become such a widely-applicable technology is due to its high accuracy without the intrusiveness of other physiological identification approaches. Unlike fingerprinting and DNA testing, users don’t need to be present or to touch anything.
Compared to iris scanners, biometric facial recognition can be used from a relative distance (as with security cameras). In this sense, facial recognition technology has all the accuracy of the other solutions without imposing on a person’s body.
How Does Biometric Facial Recognition Work?
Biometric facial recognition technologies work using pattern matching software. Human bodies have ingrained patterns that are unique to each person (fingerprints, ear shapes, hand geometry, and so on). These are known as biometric modalities.
Facial recognition works by matching the biometric modalities in an image to a person or vice versa, or from an image to another image.
The facial recognition process is broken down into five steps: detection, normalization, processing, extraction, and matching.
The face image detector locates human faces from an image, separating the face from a simple or complex background. This component of the technology divides ‘face’ and ‘non-face’ images to segment the face, its location, and its scale.
Next, the face normalization tool geometrically aligns the face using canonical coordinates. Harnessing facial landmarks, such as the eyes, ears, nose, mouth, and face outline, the normalization process picks out the identifying facial features and their positioning.
The face processing step is not always present in traditional facial recognition systems. However, in deep learning technologies, this tool will help to process the lighting and pose of the face in order to relay the two-dimensional image into a three-dimensional representation.
The extraction process pulls out facial features to differentiate between individuals. Using around 32 geometric and photometric points, this process modifies the pixels of features in an image into a vector representation (or vice versa).
The final step is the comparison stage. This is where a facial comparison software matches patterns from the image to databases to find the best match. Facial recognition software used for identification is often referred to as Automated Facial Recognition (AFR).
When comparing facial images to databases, AFR performs either closed-set or open-set identification. Closed-set identification is when a person is known to be in the database, therefore verifying and authenticating a person. This is the process used in KYC verification.
Open-set identification is when the person is not known to be in the database, as with crime database searches.
While most facial recognition software incorporates all of these stages, traditional and deep-learning systems differ in how the features are recognized.
In traditional biometric facial recognition technologies, the features are programmed by the human being designing the algorithms in the software. In a deep learning tool, the features that are extracted and compared are learned via the neural network. This neural network originally comprises a pool of subjects which are used to train the technology. This type of software expands its neural network and breadth of ‘knowledge’ with the more faces and features it analyzes.
While biometric facial recognition technology works by completing the steps above, the way in which it compares faces depends on the task in hand. Most often, facial recognition is used to identify, authenticate, or classify a person.
In the case of identification, the software will match the unique modalities of a person against many other faces to determine the individual’s identity. For verification purposes, the software will attempt to match a person’s facial features to only one person in the hope of verifying that a person is who they claim to be.
When it comes to categorization, the software matches a person’s features to a specific group to determine if a person belongs to this group (usually based on age, race, sex, etc.).
Challenges of Biometric Facial Recognition
While biometric facial recognition can be profoundly useful for identifying, verifying, and classifying human beings, it can also be easily misused. A rapidly-accelerating technology, facial recognition software comes loaded with heavy warnings as an ethical concern bubbles to the surface. Selecting the wrong digital identification and verification system for your company may lead to these challenges.
Privacy and Security
One of the major issues with biometric facial recognition software is the large-scale collection of personal biometric data. Not only is this practice morally questionable, but it also poses intense issues with data security. With the GDPR and similar legislation cracking down on data ownership, usage, and distribution, biometric facial recognition technology has received a lot of heat. While the technology itself may not breach usage rights, hacks and leaks lead to sensitive data being made public.
This was seen in the major biometric systems breach of the UK police and related defense firms in 2019, where a million people’s data was leaked.
Even more terrifying was the Sensenet data leak. On the one hand, the hack seriously impinged on the privacy of millions of people as biometric information was released. On the other hand, it showed that the Chinese government is using biometric facial recognition to track (at least) 2.6 million people. While hacks and breaches demonstrate the security issues of storing such sensitive data, this example from the Chinese government exemplifies the Orwellian issues that can occur if biometric facial recognition is abused.
Due to the fragility of this situation and to the obvious potential for misuse, the EU (in accordance with the GDPR) is making a stand on facial recognition technology by laying down strong limits as to usage rights of such data.
The first GDPR fine related to facial recognition technology was issued back in 2019. The Swedish Data Protection Authority (DAP) recently fined a Swedish school that was using AFR to monitor attendance. The DPA found the school to be processing personal data more than was necessary and without implementing effective data protection mechanisms. The DPA also claimed that the school did not have prior consent and had no legal basis for collecting such data.
Misidentification
While biometric facial recognition technology has the power to be extremely accurate, the specific technology used and the way in which it is executed can seriously affect its accuracy. This can lead to misidentification.
When applied to crime, for example, misidentification can result in the wrong person being identified as the perpetrator. This is exemplified in the case of the Welsh police, where their internal facial recognition software misidentified 2300 people as criminals. Equally, studies have shown that the face recognition service used by London’s Metropolitan police has an 81% error rate.
Fraud is another area where misidentification by facial recognition systems is a big problem. Often known as face spoofing attacks, fraudulent users will use photographs or pre-recorded videos to fool facial recognition software in order to open or access accounts, such as bank accounts or computer accounts. In fact, studies have shown that even Windows‘ own facial recognition software can be spoofed with a printed picture.
Bias
One of the inherent concerns with biometric facial recognition is bias. In traditional systems, the person writing the technology’s algorithm would decide on the features that the software would recognize. If the programmer does not give a diverse enough set of recognizable features, this skews what the software can and can’t pick up.
The problem is also present in deep-learning facial recognition software. If the original pool from which the software learns is not diverse enough, there will be bias in what the software can detect.
Due to the cultural dynamics of our society, the vast majority of programmers are white males. In the facial recognition software of today, this has manifested as a bias toward white males.
The “Gender Shades” project highlighted this issue with results that showed women of color to be the most vulnerable group to gender misclassification. In fact, the study showed that the misidentification rate for darker-skinned females was 34.4% higher than that of light-skinned males.
This is a huge problem when it comes to racial prejudice, especially in terms of criminal proceedings. In a study that looked into Amazon’s facial recognition tool, “Rekognition,” racial bias was illuminated clearly. When 535 members of US Congress were compared with a database of 25,000 arrest photos, the system returned 28 false positives. While a 5% error rate doesn’t seem awful, the problem lies in the fact that 39% of those false matches were people of color, while people of color only made up 20% of the original group. This demonstrates an unquestionable racial bias in this particular facial matching software.
Real-World Use Cases of Biometric Facial Recognition
Currently, there are three major markets in which biometric facial recognition technology is being applied: security, authentication (identification and verification), and analysis.
Across industries, these three applications are being exercised in the following innovative ways.
Biometric facial recognition systems are being utilized by criminal justice systems all over the world. One of the primary applications of the technology, widely used in China and the UK, is to track criminals and identify fugitives. It is also being used to trace missing adults and children through private and public security cameras to reunite them with their families. This initiative crosses over with the fight against human trafficking, where biometric facial recognition is being used to scan millions of online adverts to find victims.
As with the case of GetID, biometric facial recognition technology is also proving itself as an excellent fraud prevention tool, helping banks and financial institutions to weed out fraudsters. Where previously fraudulent users would submit false photographs, they must now pass biometric systems. These are much harder to fool.
In terms of e-commerce, the possibilities for applying biometric facial recognition technology are endless. Beijing KFC has been experimenting with one application of the technology to improve service. The system remembers customer orders along with a biometric record of their face. On the customer’s next visit, the technology will recognize their face and suggest their previous order.
Facial recognition technology is also being used to disrupt payment systems, as can be seen with AliPay. AliPay has rolled out the world’s first system, where users pay through facial recognition. This speeds up the payments process as well as adding an extra layer of security, again improving customer service.
In the transport sector, the technology is being used all over the world to ease wait times for customers. Delta Air Lines, for example, is trialing facial recognition scans in airports to speed check-in times. Elsewhere in the world, China is attempting to reduce queue time in subways with facial recognition. Germany is executing a similar pilot program to do the same in railway stations.
The healthcare industry is also introducing biometric facial recognition for a number of revolutionary industry changes. Face comparison software is being employed in patient monitoring and sentiment analysis. This helps healthcare professionals to determine if patients are comfortable and if they need pain relief. Interestingly, the technology is also being used to detect rare genetic disorders, such as Cornelia de Lange syndrome and Angelman syndrome. Similarly to the banking sector, the healthcare industry is also utilizing biometric facial technology as a fraud prevention measure.
As mentioned above, facial recognition software is also being introduced in the education sector. While some of the first applications of this technology as an attendance tracking tool haven’t been successful due to data protection issues, this idea is still on the table for many countries. Equally, schools are considering the use of facial recognition to safeguard children against bullying.
Another interesting use case for facial recognition technology is to help blind people better function in social situations. By recognizing facial expressions, the software can buzz blind users to let them know other people are smiling!
How is GetID Leveraging Biometric Facial Recognition?
GetID is using biometric facial recognition technology as a way to help companies and financial institutions identify and verify their users, while also helping those users identify themselves more easily online.
Our facial recognition service automatically identifies and verifies users by matching their image to their official documentation and existing databases. This will highlight any fraudsters, Politically Exposed Persons, or individuals subject to sanctions. This speeds up and cuts costs in your KYC process while ensuring complete AML compliance.
What makes GetID’s application of facial recognition so promising is that we combine it with various identification and verification procedures to increase security and accuracy. Where other applications of facial recognition softwares only offer a single layer of verification, GetID guarantees security with our multidimensional authentication process. Our biometric facial recognition system is teamed with Optimal Character Recognition and Biometric Liveness Detection. This creates a multilayered approach that verifies users most accurately, safeguarding against face spoofing and misidentification.
Not only does this speed up the process of KYC for many organizations, but it also enhances precision and removes human error in the identification and authentication process.
Conclusion
Harnessing biometric facial recognition tools for identification and verification can help to speed up your KYC processes and ensure that you are up-to-date with AML compliance. However, deploying the wrong software can leave you facing issues of data security and misidentification.
Thankfully, GetID’s automated multilayered verification platform provides a biometric identity authentication technology that overcomes these challenges. Combining biometric facial recognition with liveness detection, GetID’s identity verification solution offers top-notch accuracy for speedier, more cost-effective verification. Simultaneously, our data storage architecture keeps all biometric data firmly locked away with the user.
For your company, this means full data security and AML compliance, with sleeker KYC processes. For your customers, this means smoother onboarding and security they can trust.
If you’re looking for a robust biometric identification and authentication solution to streamline your KYC and AML processes, contact GetID today to learn more.